Artificial intelligence (AI) can do a plethora of astonishing things in the medical space, from automating triage and administrative tasks to assisting in mental health support and medical image analysis. On top of these, every now and then, AI makes curious medical discoveries, detecting things that – to the best of our human knowledge – should not be detectable from the input data.
These unusual associations present brand-new challenges to medical professionals who need to better understand how smart algorithms come to such conclusions that have eluded humans for decades. In this article, we consider some striking examples of AI finding connections that would otherwise remain invisible to human experts. Such observations highlight the technology’s potential and how it will continue to surprise us in the years to come.
Debiasing and speeding up radiological imaging
With the majority of FDA-approved medical AI tools targeted at radiological use, it is not surprising that the technology has found unusual associations in this field.
In an interesting study, MIT scientists showed that deep learning algorithms can predict the self-reported race of patients from radiological images alone. This is a feat even the most seasoned physicians cannot do, and it’s not clear how the model was able to do this. Such insights can have practical uses as they help to counter bias inherent in medical records.
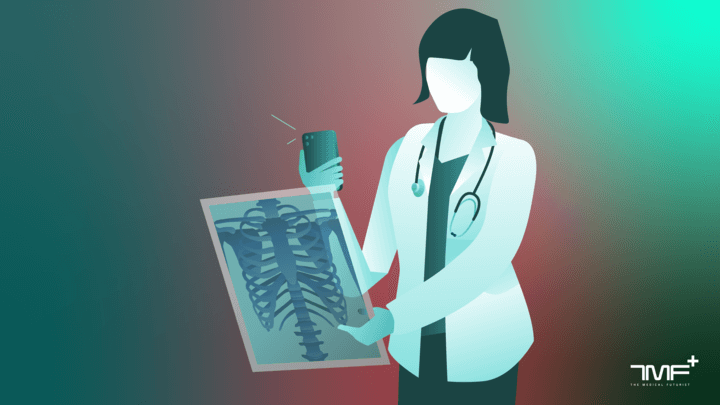
At UMass Memorial Health, at least 40 AI tools assist in clinical workflows, handling tasks such as getting results to critical patients faster and assisting in billing. They also aid in refining the quality of radiological images. This has been associated with patients spending less time in MRI machines. The scanning process is thus made more tolerable and patients feel less anxious.
“MRIs are long, uncomfortable and loud, but they’re really valuable for medical decision making,” Dr. Elisabeth Garwood from UMass Memorial Health explained. “The acceleration algorithms at UMass are making our MRIs 25 percent faster, and that really hacks the patient experience that they’re in the MRI for less time.”
Enhancing diagnoses with photos, voice recordings and breath scans
In the digital health market, digital biomarkers, or digital data that provide insights into an individual’s health status, are gaining popularity, but AI seem to be able to derive insights from its own unusual sources.
Researchers trained a model to analyse 10-second-long voice recordings to diagnose type 2 diabetes based on certain acoustic features. While not displaying ideal performance, the model produced promising results, which were better than chance in correctly identifying diabetic individuals. This development makes the scenario of being able to detect one’s blood glucose levels from a smartphone quite plausible.
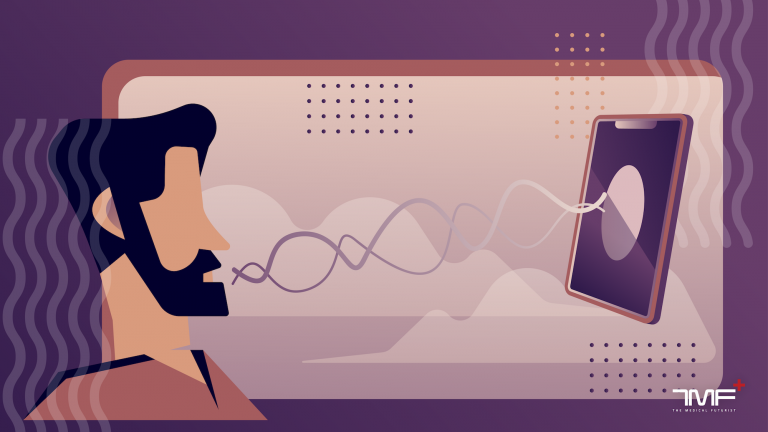
Dr. Jude Kong, who leads the Africa-Canada AI & Data Innovation Consortium and the Global South AI for Pandemic & Epidemic Preparedness & Response Network, has been collaborating with governments to employ bespoke AI tools for practical diagnoses through unconventional means.
For example, a model deployed in Ethiopia can help determine if a patient’s paralysis is indicative of polio based on a photograph. In Peru, they co-created a breathalyzer that leverages AI technology to help diagnose respiratory disease.
Google researchers also employed AI to detect health risks from images. In particular, they trained deep-learning models to identify signs indicating long-term cardiovascular risks from retinal images.
Traditionally, in order to assess those risks, doctors need to manually look at the retina, do blood tests and consider other factors like age and BMI. Impressively, the AI taught itself what to look for in retinal images alone after having gone through enough data to identify patterns found in the eyes of people at risk.
Such technology can prove to be lifesaving, especially considering the fact that some 17 million people die of cardiovascular diseases every year. It can help doctors and even patients run a quick screening test and assess their risk and take subsequent preventive actions.
Improving psychiatric care with brain waves, early Alzheimer’s detection and coma recovery assessments
Psychiatric care stands to gain a boost thanks to the assistance of AI. As surprising as it might sound, treatment selection for antidepressants is generally based on trial and error. This is the reason that only 30% of patients respond well to the first antidepressant prescribed, but the input of AI can provide a more effective method.
By studying the brainwaves of patients, researchers used a machine learning algorithm to identify the best antidepressant: sertaline, in this case. Their results showed that 65% of patients with a particular brainwave pattern indicated a strong response to sertraline. One of the researchers suggested that this method is “far better” than relying on clinical factors, such as certain symptoms, to try to guess whether a drug will have a favourable effect on patients.
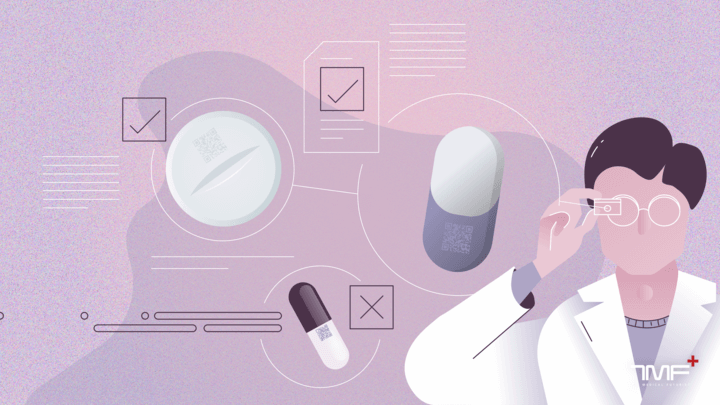
For a condition like Alzheimer’s, patients are commonly diagnosed with the condition after the symptoms manifest. These can be very debilitating, such as memory loss, personality changes and depression. A research team at the University of California in San Francisco trained an algorithm to look for indicative signs of Alzheimer’s from another angle.
The researchers trained a deep learning algorithm on FDG-PET scans, a method used to study the metabolic activity of brain cells. This taught the AI to recognise metabolic patterns associated with Alzheimer’s disease. In subsequent tests, the AI detected the condition with 100% sensitivity, on average more than six years prior to the final diagnosis!
Being in a coma or vegetative state can be one of the most ethically-taxing issues in healthcare. Based on doctors’ recommendations, relatives of such patients can decide if they would like to terminate life support. It’s a highly debatable issue what the decision will prolong: the patient’s life or suffering, while also costing both the relatives and the healthcare system. However, AI can aid in making more informed decisions in these cases, correctly predicting if one will regain consciousness even after doctors conclude an unlikely recovery.
Such an AI system has been developed by the Chinese Academy of Sciences and PLA General Hospital in Beijing. Their algorithm reportedly achieved about 90 percent accuracy on prognostic assessments. The software analyzes brain scans to re-evaluate doctor’s decisions. In at least 7 cases where doctors were confident that patients wouldn’t regain consciousness, the AI contradicted them and indeed those patients woke up within 12 months of the brain scans. “Our machine can ‘see’ things invisible to human eyes,” Dr Song Ming, first author of the study, said.
This is because the evaluation of patients is done using a brain scan with functional magnetic resonance imaging and the rapidly evolving neural activities can prove challenging for doctors to detect. On the other hand, a machine learning algorithm can detect minute changes indicative of an ongoing recovery. This could help doctors and relatives make more informed decisions when it comes to such patients.
Aiding the identification and treatment of rare diseases
If a disease is rare, then its identification and treatment will pose a challenge. Yearly, about half a million children are born with a rare hereditary disease around the world. However, many of these cases present with specific physical features that can help in their identification. Clinicians might miss these due to the fact that they’ve never seen such cases. In addition, due to the rarity of such cases, treatment options are often poorly understood. However, nothing escapes the meticulous eye of AI.
Researchers from the University of Pennsylvania used a predictive AI tool to identify a suitable medicine to save the life of a patient with idiopathic multicentric Castleman’s disease (iMCD). This rare condition is characterised by a poor survival rate and a lack of treatment.
But after analysing thousands of existing medications, the AI system predicted that an FDA-approved monoclonal antibody used to treat other conditions would likely work for iMCD; and it did. The patient is now almost two years into remission, and this approach could also be applicable to other rare diseases.
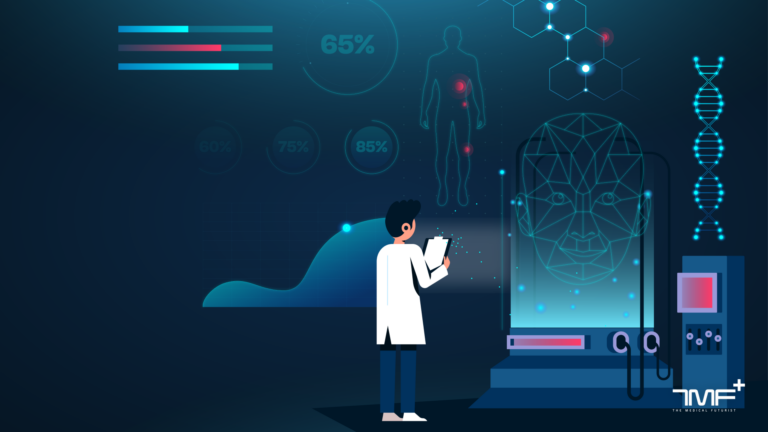
Researchers based in Germany developed and trained an algorithm to help the identification of diseases caused by a change in a single gene. These include conditions like mucopolysaccharidosis, Mabry syndrome and Kabuki syndrome, where those affected have characteristic facial features.
The researchers trained the neural network DeepGestalt with 30,000 portrait photos of those with such rare conditions. “In combination with facial analysis, it is possible to filter out the decisive genetic factors and prioritize genes,” said Prof. Krawitz who worked on this study. “Merging data in the neuronal network reduces data analysis time and leads to a higher rate of diagnosis.”
Their results showed that with the help of AI, identifying rare diseases was much more accurate. Using this technique could fast-track the identification and treatment of those affected from early on.
Synchronising surgical rooms
While there is a promising technological future of surgery, the focus is mostly on assisting surgeons instead of the whole surgical team working behind the scenes of procedures. To enhance the collaboration and synchronicity of surgical teams, startup eXeX has developed a dedicated AI platform. It leverages the Apple Vision Pro headset to improve communication, clarity and orientation within the surgical suite.
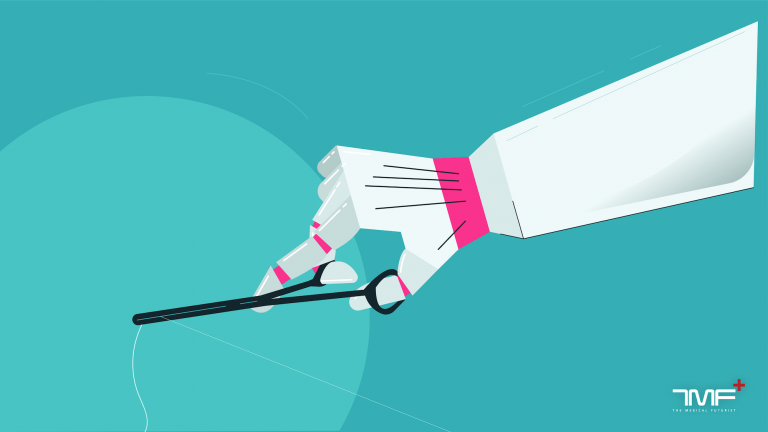
Their product combines a language model with a computer vision model to assist the surgical teams in answering questions during a procedure and help them orient themselves in the room.
“The app running on the headset has a full spatial awareness in the room in real time, and it understands exactly where the user is and even knows what the user is looking at,” explained Nicholas Cambata, COO of eXeX. For example, a surgical assistant could set up a tray prior to a procedure and request a check from the AI. The tool can identify any missing equipment and guide the user to the exact location in the room.
Points system to assess one’s need for hospitalization
This was the premise of a pilot project from Bering Research and GPs at Axbridge Surgery in Somerset, England. An algorithm was deployed to predict which patients might need to be admitted to a hospital and to help GPs work on reducing the risk.
The AI allocates points, based on a percentage scale, according to underlying health conditions and contributing factors like elevated blood pressure or smoking habits. The higher the points, the more likely the patient will need hospitalization.
The aim is to have GPs intervene earlier, make accurate predictions on hospital admissions, and help hospitals plan on allocating their resources.
While these unusual associations give a glimmer of hope to millions of patients around the world, we must be cautious about how we take this news. The experiments conducted need to be validated and repeated on a larger scale while considering other contributing factors like comorbidities.
However, it does show that artificial intelligence can become an integral part of not only treating patients but also identifying risks, and taking preventive measures we have never thought about before.
The post AI’s Unforeseen Medical Discoveries: The Curious Case Of Unusual Associations appeared first on The Medical Futurist.